This article is part of ArangoDB’s open-source performance benchmark series. Since the previous post, there are new versions of competing software on which to benchmark. Plus, there are some major changes to ArangoDB software. For instance, in latest versions of ArangoDB, an additional storage engine based on Facebook’s RocksDB has been included. So we waited until its integration was finished before conducting a new benchmark test. Besides all of these factors, machines are now faster, so a new benchmark made sense.
Every time you move your Mac laptop—from the office to home, from home to the neighborhood coffee shop—you must adjust its network settings.
- The switch operates at layer 2 of the OSI model and therefore uses the MAC or Ethernet address for making decisions for forwarding data packets. The switch monitors data traffic on its ports and collects MAC address information in the same way the bridge does to build a table of MAC addresses for the devices connected to its ports.
- Juniper Networks EX Series Ethernet Switches store MAC addresses in the Ethernet switching table, also called the MAC table. When the aging time for a MAC address in the table expires, the address is removed. If your switch runs Juniper Networks Junos operating system (Junos OS) for EX Series.
Before I get into the benchmark specifics and results, I want to send a special thanks to Hans-Peter Grahsl for his fantastic help with MongoDB queries. Wrapping my head around the JSON notation is for sure not impossible, but, boy, can querying data be complicated. Thanks Hans-Peter for your help! Also a special thanks to Mark, Michael and Jan from our team for their excellent and tireless work on this benchmark. Great teamwork, crew! After we published the previous benchmark, we received plenty of feedback from the community — thanks so much to everyone for their help, comments and ideas. We incorporated much of that feedback in this benchmark.
For instance, this time we included the JSONB format for PostgreSQL. ArangoDB, as a native multi-model database, competes with many single-model storage technologies. When we started the ArangoDB project, one of the key design goals was and still is to at least be competitive with the leading single-model vendors on their home turf. Only then does a native multi-model make sense. To prove that we are meeting our goals and are competitive, we run and publish occasionally an update to the benchmark series. For comparison, we used three leading single-model database systems: Neo4j for graph; MongoDB for document; and PostgreSQL for relational database.
Additionally, we benchmarked ArangoDB against a multi-model database, OrientDB. Of course, performing our own benchmark can be questionable. Therefore, we have published all of the scripts necessary for anyone to repeat this benchmark with minimum effort. They can be found here on Github:. We used a simple client/server setup. We used the following instances:. Server: i3.4xlarge on AWS with 16 virtual cores, 122 GB of RAM.
Client: c3.xlarge on AWS with four virtual CPUs, 7.5 GB of RAM and a 40 GB SSD The setup costs 35 US dollars a day. To keep things simple and easily repeatable, all products were tested as they were when downloaded. So you’ll have to use the same scripts and instances if you want to compare your numbers to ours.
We used the latest GA versions (as of January 26, 2018) of all database systems and not to include the RC versions. Below are a list of the versions we used for each product:. Neo4j 3.3.1. MongoDB 3.6.1. PostgreSQL 10.1 (tabular & jsonb). OrientDB 2.2.29.
ArangoDB 3.3.3 For this benchmark we used NodeJS 8.9.4. The operating system for the servers was Ubuntu 16.04, including the OS-patch 4.4.0-1049-aws — this includes Meltdown and Spectre V1 patches. Each database had an individual warm-up. Descriptions of Tests We use this benchmark suite internally for our own assessment, our own quality control, to see how changes in ArangoDB affect performance. Our benchmark is completely open-source.
You can download all of the scripts necessary to do the benchmark yourself in our. The goal of the benchmark is to measure the performance of each database system when there is no query cache used. To be assured of this, we disabled the query cache for each software that offered one. For our tests we ran the workloads twenty times, averaging the results.
Each test starts with an individual warm-up phase that allows the database systems to load data in memory. For the tests, we used the. It contains 1.6 million people (vertices) connected via 30.6 million edges. With this dataset, we can do basic, standard operations like single-reads and single-writes, but also graph queries to benchmark graph databases (e.g., the shortest path). The following test cases have been included, as far as the database system was capable of performing the query:. single-read: these are single document reads of profiles (i.e., 100,000 different documents).
single-write: these are single document writes of profiles (i.e., 100,000 different documents). single-write sync: these are the same as single-writes, but we waited for fsync on every request. aggregation: these are ad-hoc aggregation over a single collection (i.e., 1,632,803 documents). We computed the age distribution for everyone in the network, simply counting how often each age occurs. neighbors second: we searched for distinct, direct neighbors, plus the neighbors of the neighbors, returning ID’s for 1,000 vertices.
neighbors second with data: we located distinct, direct neighbors, plus the neighbors of the neighbors and returned their profiles for 100 vertices. shortest path: this the 1,000 shortest paths found in a highly connected social graph. This answers the question how close to each other two people are in the social network. memory: this is the average of the maximum main memory consumption during test runs. The throughput measurements on the test machine for ArangoDB — with RocksDB as storage engine — defined the baseline (100%) for the comparisons. Lower percentages indicate a higher throughput. Accordingly, higher percentages indicate lower throughput.
Overall Results The graph below shows the overall results of our performance benchmark. In the sub-sections after this graph, we provide more information on each test. Appendix – Details about Data, Machines, Products and Tests For this NoSQL performance benchmark, we used the same data and the same hardware to test each database system. If you want to check or understand better our results, in this appendix we provide details on the data, the equipment, and the software we used. We also provide more details on the tests we performed, as well as describe some of the adjustments made to accomodate the nuances of some database systems. Data Pokec is the most popular online social network in Slovakia.
We used a snapshot of its data provided by the Stanford University SNAP. It contains profile data from 1,632,803 people. The corresponding friendship graph has 30,622,564 edges. The profile data contain gender, age, hobbies, interest, education, etc. However, the individual JSON documents are very diverse because many fields are empty for many people. Profile data are in the Slovak language. Friendships in Pokec are directed.
The uncompressed JSON data for the vertices need around 600 MB and the uncompressed JSON data for the edges require around 1.832 GB. The diameter of the graph (i.e., longest shortest path) is 11, but the graph is highly connected, as is normal for a social network. This makes the shortest path problem particularly hard. Hardware All benchmarks were done on a virtual machine of type i3.4xlarge (server) on AWS with 16 virtual cores, 122 GB of RAM and a 1900 GB NVMe-SSD. For the client, we used a c3.xlarge on AWS with four virtual CPUs, 7.5 GB of RAM and a 40 GB SSD. Software We wanted to use a client/server model for the benchmark. For this, we needed a language to implement the tests.
Therefore, we decided that it has to fulfill the following criteria:. Each database in the comparison must have a reasonable driver. It’s not one of the native languages our contenders has implemented. This would potentially give an unfair advantage for some. This ruled out C and Java. The language must be reasonably popular and relevant in the market.
The language should be available on all major platforms. This essentially left JavaScript, PHP, Python, Go and Ruby. We decided to use JavaScript with node.js 8.9.4. It’s popular and known to be fast, in particular with network workloads. For each database we used the most up-to-date JavaScript driver that was recommended by the respective database vendor. We used the following Community Editions and driver versions:.
ArangoDB V3.3.3 for x8664 (arangojs@5.8.0 driver). MongoDB V3.6.1 for x8664, using the WiredTiger storage engine (mongodb@3.0.1 driver). Neo4j V3.3.1 running on openjdk 1.8.0151 (neo4j@1.5.3 driver). OrientDB 2.2.29 (orientjs@2.2.7 driver).
PostgreSQL 10.1.1 (pg-promise@7.4.1 driver) All databases were installed on the same machine. We did our best to tune the configuration parameter. For example, we switched off transparent huge pages and configured up to 60,000 open file descriptors for each process. Furthermore, we adapted community and vendor provided configuration parameters from Michael Hunger of Neo4j and Luca Garulli of OrientDB to improve individual settings. Tests We made sure for each experiment that the database had a chance to load all relevant data into RAM. Some database systems allow explicit load commands for collections, while others do not.
Therefore, we increased cache sizes where relevant and used full collection scans as a warm-up procedure. We didn’t want to benchmark query caches or likewise — a database might need a warm-up phase, but you can’t compare databases based on cache size and efficiency. Whether a cache is useful or not depends highly on the individual use case, executing a certain query multiple times.
For single document tests, we used individual requests for each document, but used keep-alive and allowed multiple simultaneous connections. We did this since we wanted to test throughput rather than latency. We used a TCP/IP connection pool of up to 25 connections, whenever the driver permitted this. All drivers seem to support this connection pooling, except Neo4j. We sent instead twenty-five requests via NodeJS to Neo4j. Single Document Reads (100,000 different documents) In this test we stored 100,000 identifiers of people in the node.js client and tried to fetch the corresponding profiles from the database, each in a separate query. In node.js, everything happens in a single thread, but asynchronously.
To load fully the database connections, we first submitted all queries to the driver and then waited for all of the callbacks using the node.js event loop. We measured the wallclock time from just before we started sending queries until the last answer arrived. Obviously, this measures throughput of the driver and database combination and not latency.
Therefore, we gave as a result the complete wallclock time for all requests. Single Document Writes (100,000 different documents) For this test we proceed similarly: We loaded 100,000 different documents into the node.js client and then measured the wallclock time needed to send all of them to the database, using individual queries. We again first scheduled all requests to the driver and then waited for all callbacks using the node.js event loop.
As above, this is a throughput measurement. Single Document Writes Sync (100,000 different documents) This is the same as the previous test, but we waited until the write was synced to disk — which is the default behavior of Neo4j. To be fair, we introduced this additional test to the comparison. Aggregation over a Single Collection (1,632,803 documents) In this test we did an ad-hoc aggregation over all 1,632,803 profile documents and counted how often each value of the AGE attribute occured. We didn’t use a secondary index for this attribute on any of the databases.
As a result, they all had to perform a full collection scan and do a counting statistics. We only measured a single request, since this is enough to get an accurate measurement.
The amount of data scanned should be more than any CPU cache can hold. We should see real RAM accesses, but usually no disk accesses because of the above warm-up procedure. Finding Neighbors and Neighbors of Neighbors (distinct, for 1,000 vertices) This was the first test related to the network use case. For each of 1,000 vertices we found all of the neighbors and all of the neighbors of all neighbors. This requires finding the friends and friends of the friends of a person and returning a distinct set of friend ID’s. This is a typical graph matching problem, considering paths of length one or two. For the non-graph database MongoDB, we used the aggregation framework to compute the result.
In PostgreSQL, we used a relational table with id from and id to, each backed by an index. In the Pokec dataset, we found 18,972 neighbors and 852,824 neighbors of neighbors for our 1,000 queried vertices. Finding Neighbors and Neighbors of Neighbors with Profile Data (distinct, for 100 vertices) We received feedback from previous benchmarks that for a real use case we need to return more than ID’s. Therefore, we added a test of neighbors with user profiles that addresses this concern and returns the complete profiles. In our test case, we retrieved 84,972 profiles from the first 100 vertices we queried. The complete set of 853,000 profiles (1,000 vertices) would have been too much for nodejs.
Finding 1000 Shortest Paths (in a highly connected social graph) This is a pure graph test with a query that is particularly suited for a graph database. We asked the databases in 1000 different requests to find the shortest path between two given vertices in our social graph. Due to the high connectivity of the graph, such a query is hard, since the neighborhood of a vertex grows exponentially with the radius. Shortest path is notoriously bad in more traditional database systems, because the answer involves an a priori unknown number of steps in the graph, usually leading to an a priori unknown number of joins.
Nuances The section above describes the tests we performed with each database system. However, each has some nuances that required some adjustments. One cannot always in fairness leave all factors constant. ArangoDB ArangoDB allows you to specify the value of the primary key attribute key, as long as the unique constraint is not violated. It automatically creates a primary hash index on that attribute, as well as an edge index on the from and to attributes in the friendship relation (i.e., the edge collection).

No other indexes were used. MongoDB Since MongoDB treats edges just as documents in another collection, we helped it a bit for the graph queries by creating two more indexes on the from and to attributes of the friendship relation. For MongoDB, we had to avoid the $graphlookup operator to achieve acceptable performance. We tested the $graphlookup, but performance was so slow that we decided not to use it and wrote the query in the old way, as suggested by Hans-Peter Grahsl. We didn’t even try to do shortest paths.
Please note that as the stats for MongoDB worsened significantly in comparison to what we measured in 2015, we reran the test for MongoDB with the same NodeJS version that we used in the 2015 benchmark. Results for single-reads and single-writes were slightly better with the old NodeJS version, but with no effect on the overall ranking. Since we tested the latest setup for all products, we didn’t publish the results. Neo4j In Neo4j, the attribute values of the profile documents are stored as properties of the vertices. For a fair comparison, we created an index on the key attribute. Neo4j claims to use “index-free adjacency” for the edges.
So we didn’t add another index on edges. OrientDB For OrientDB, we couldn’t use version 2.2.31, which was the latest one, because a bug in version 2.2.30 in the shortestpath algorithms hindered us to do the complete benchmark. We and the OrientDB team fixed it immediately but the next maintenance release was published after January 26. Please note that if you are doing the benchmark yourself and OrientDB takes more than three hours to import the data, don’t panic.
We experienced the same. PostgreSQL (tabular & JSONB) We used PostgreSQL with the user profiles stored in a table with two columns, the Profile ID and a JSONB data type for the whole profile data. In a second approach, for comparison, we used a classical relational data modelling with all profile attributes as columns in a table.
Resources and Contribution All code used in these tests can be downloaded from our Github repository. The repository contains all of the scripts to download the original data set, and to prepare it for all of the databases and import it. We welcome all contributions and invite you to test other databases and other workloads. We hope you will share your results and experiences.
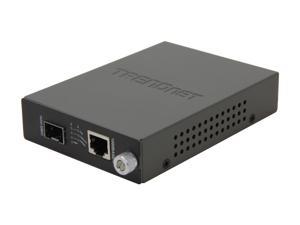
Frustrated with U-verse wireless speeds, so trying to hook up directly with one of my laptops to the U-verse modem. (Signal fluctuates from as low as 200 Kbps to close to 3Mbps.
Very inconsistent, to the point I lose connection often. Tried everything with help from AT&T Techs, but still frustrated.) Direct hookup on RoadRunner and DSL were simple. Just hook the two ends up and go. Not so with U-verse. Network looks active (green signal on laptop), but unable to display any pages on browser. Network diagnostics trouble-shooter not helpful.
Don't want to spend another two hours with tech support on phone. How do you configure Windows and/or OS for direct cable from laptop to modem?
Kindest Regards, Surferdad. I used to have the 'green' ethernet light on the computer port w/ATT DSL (w/a 'connection' manager), when I got Uverse, now the 'orange' lite is on the computer port (no 'connection' manager). It should be connecting w/the 'local area connection' lower right in your task bar, anything else, it's wrong.
More info from you needed, any old 'connection managers ' that are running trying to connect? If, so they are screwing things up, no sign in required once Uverse is installed, unlike regular DSL where sign in is required often. Good luck Chris Please NO SD stretch-o-vision or 480 SD HD Channels 1-800-983-2811 to avoid Mr. Voice Recognition I Call It Like I See It, Your Results May Vary, In My Humble Opinion Simply a U-verse user, nothing more.
Thanks, must be some 'leftover' configurations from using DSL. Can't seem to get past it with either the OS or Windows based laptops.
With Roadrunner, just plugged in and voila. Is there a setup disk or downloadable file available to enable the LAN cable hookup? Could the modem port (labeled Broadband) not be setup? The network indicates that it is connected on both systems. Just keep getting the orange light on the laptops.
Average Dating Time To Engagement Ethernet Hookup For Mac Pro
Can't seem to get to any user input that allows me to enter the modem password. Thanks again, milbrnsurg. MCP wrote: On my U-verse RG, the port labeled Broadband is not used. All my computers are plugged into the ports labeled Local Ethernet.
I think the Broadband port is an input to the RG, possibly for fiber-to-the-premises (FTTP) configurations. Absolutely correct! Plug your computers into the yellow Local Ethernet ports. I wish I had something plugged into my broadband port. That would me that I would have. Oh never mind.